
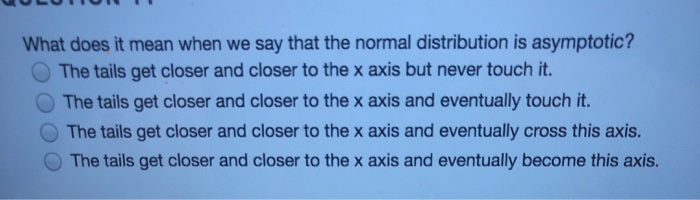
Like with the Cauchy the median exists, as would the median absolute deviation as a measure of scale.Bleed-through (often termed crossover or crosstalk) of fluorescence emission, due to the very broad bandwidths and asymmetrical spectral profiles exhibited by many of the common fluorophores, is a fundamental problem that must be addressed in both widefield and laser scanning confocal fluorescence microscopy. If it's a t-distribution with 2 degrees of freedom, the first moment exists, but the second does not, so as with the Cauchy distribution, there would be no meaningful estimate of standard deviation, and therefore, you would not be able to compute the standard confidence interval. For the Cauchy, the median does exist though, and you could compute the median absolute deviation as a measure of scale. That's the Cauchy distribution, which has no moments, so you would not be able to compute the mean, standard deviation or the standard confidence interval. The worst case would be the t-distribution with 1 degree of freedom. But when you have few degrees of freedom, the moments based estimators (mean, variance, skewness, and kurtosis, for instance) can be biased by outliers.

If you have many degrees of freedom, you can compute the standard confidence intervals. In short, there's probably no straightforward answer to your question.Ĭaroline, I think it depends on which distribution within the t- family you're using (i.e., the number of degrees of freedom). Still another approach, which is hardly used would be two assume asset returns follow a mixture distribution (e.g., 90% of the time it's a normal with mean = mu, and standard deviation = 5, 10% of the time, it's a normal with mean mu, and standard deviation = 25). Also, some people assume time-varying parameters, instead of the fixed parameters (e.g., the GARCH family for time-varying volatility). With long-range dependence, shocks can take a long time to die out. However, there are other features of financial asset return that the t-distribution (no matter how many degrees of freedom you find) will not capture, such as long-range dependence, which nullifies iid. You could use quantile-quantile plots to get a visual sense of how many degrees of freedom work best. I think with asset returns, you could model them as a t-distribution, maybe with somewhere between 5-10 degrees of freedom, which is very far from what the normal distribution would suggest, since extreme events are likely in the real world, while with the normal distribution 99% of all observations will lie within 3 standard deviations of the mean (Chebyshev's theorem). Also, the number of moments that exists equals the degrees of freedom minus one that's why the Cauchy has no moments. Standard t-distributions includes as special cases the Cauchy (when you have 1 degree of freedom), and the normal is a t-distribution with infinite degrees of freedom. The t-distribution is a family of distributions typically defined by the degrees of freedom parameter (a non-central t-distributions also exists to reflect skewness). You might want to have a look at Evans, Hastings, and Peacock's book "Statistical Distributions".
